Design rules improve the efficiency, specificity and functionality of gene knockout
Although CRISPR-Cas9 has become widely used for targeting specific genes for loss-of-function studies, the effectiveness of guide RNA reagents at creating double-strand breaks (DSBs) that result in a functional protein knockout depend heavily on the design of the guide RNAs. Using guide RNAs that have been designed based on an algorithm trained to score and select those RNAs that will give functional, specific knockout can make the difference between a conclusive experiment or a confounding one.
We have compiled a list of the top 5 reasons to use algorithm-designed guide RNAs, like the Dharmacon™ Edit-R™ product lines, to ensure the best chance of a successful knockout experiment.
-
Increased efficiency of creating DSBs
If you only use the “early exon” rule to design a guide RNA, how many more cells will you have to sort through and characterize in order to find one with the edit you need? Higher efficiency of editing means less work downstream; less work means faster results with lower total expenditure.The data below demonstrate that the guide RNAs that have high functionality scores from our Edit-R algorithm have correspondingly higher editing efficiency (shown by NGS analysis) than lower scoring designs.
-
Higher likelihood of functional gene knockout
Not all DSBs result in imperfect repair that leads to functional gene knockout. The data below demonstrates that although the indicated early-exon guide RNAs gave good indel formation (A), a secondary start site enabled the gene to produce a functional protein as demonstrated by the lack of GFP signal when these guide RNAs were used in a GFP reporter assay (B). GFP signal would indicate disruption of the functional protein, and these guide RNAs (indicated by red circle) did not induce a phenotype. -
Better specificity checking = avoidance of off-targets
Not all online gRNA design tools incorporate equivalent alignment methods to identify designs that will only target the site of interest. While nearly all online tools will identify potential off-targets with a perfect match or 1-3 mismatches, the Edit-R alignment tool is the only one that will also identify off-targets due to gaps or bulges between the guide RNA and the DNA target. If the tool cannot predict an off-target (and avoid it) how can it be specific and the user confident in the results? The Edit-R algorithm reduces the chance of off-targets with more specific designs from more thorough alignment analysis.
-
No design step required = ease of use and flexibility of format, scale and scope for your experiment
Instead of having to use a third party tool to design your guide RNAs, potentially using some guesswork as to which ones you should choose, with Edit-R predesigned guide RNAs you simply search for your gene and add the products to your cart. No extra steps, no custom orders, just access to highly efficient, specific, and functional crRNAs or sgRNAs.Since the Edit-R algorithm allows for the predesign of crRNAs and sgRNAs across the genomes of human, mouse and rat gene, we are able to offer a wide range of formats and scales for your gene editing experiment. Simply search for your gene of interest to get started! Working with more than a few genes? No problem. Browse our predefined mouse and human libraries or assemble a customized cherry-pick collection with your own gene list.
-
Get great gene knockout, regardless of the guide RNA format
The rules developed to select highly functional guide RNAs can be applied to both synthetic crRNAs and expressed sgRNAs, so regardless of which product format is best suited to your experiment and your cell type, you can be confident that the Edit-R predesigned guide RNA of choice will have all of the advantages of the Edit-R algorithm.
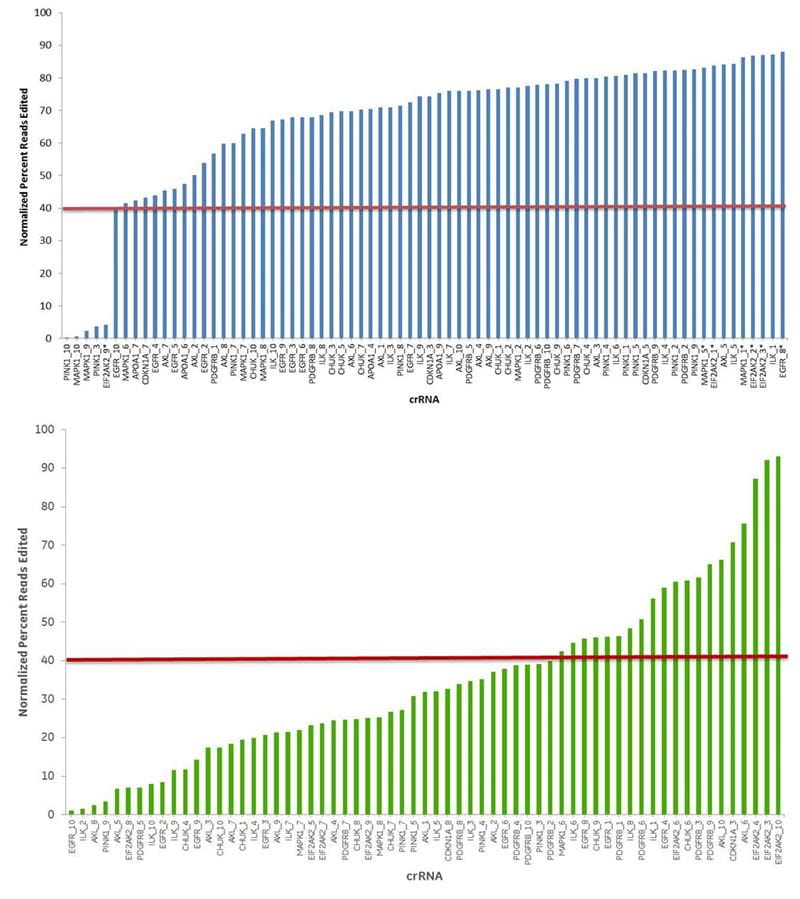
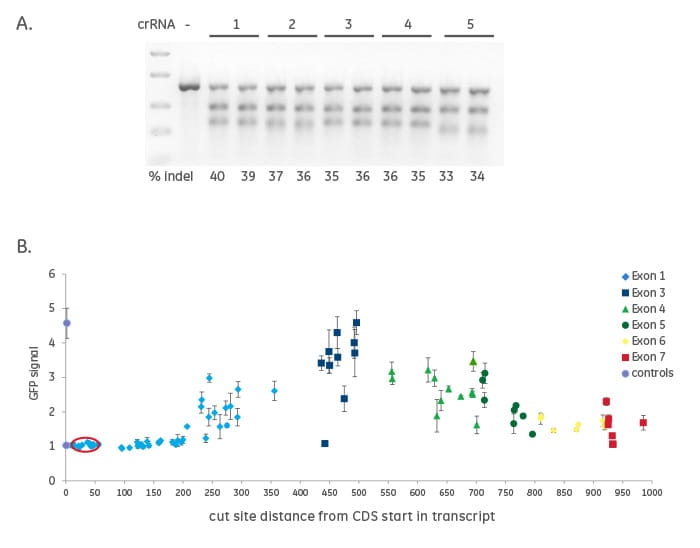
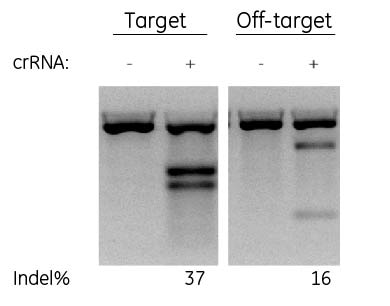
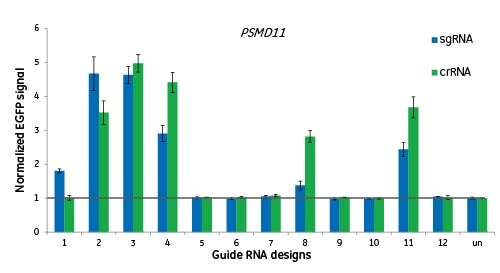
Additional Resources
CRISPR-Cas9 Gene Editing Products
- Optimized tools for high-confidence genome engineering
An algorithm for selecting functional and specific CRISPR-Cas9 guide RNAs - Poster
- Review our poster on selection of optimal CRISPR-Cas9 reagents for improving experimental outcomes
Improve CRISPR-Cas9 experiments with rationally designed guide RNAs - Webinar
- CRISPR-Cas9 gene editing is a powerful technique that enables gene knockouts with greater speed and accuracy than previous approaches. Dharmacon has developed key technologies to improve CRISPR-Cas9 mediated gene knockout.